We closed this fantastic year with our 2023 partner's meeting.…
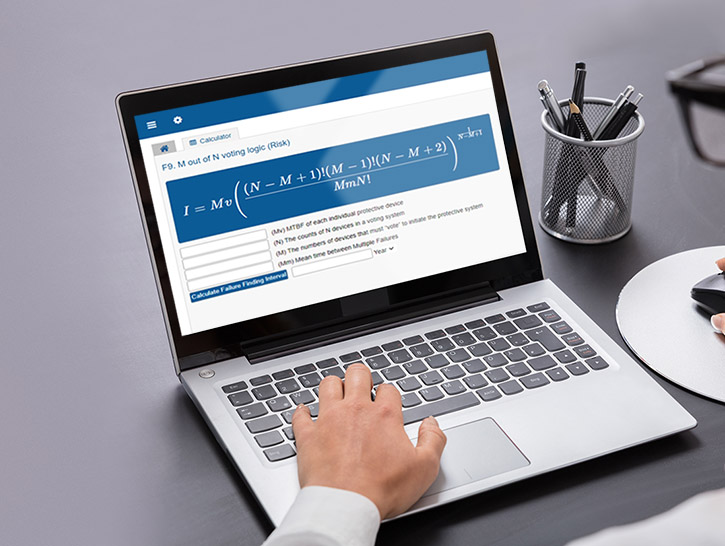
Reliability Engineering Applied to Maintenance (REAM)
Suppliers and users of any product want that it performs well during its lifetime. That is, the item must perform within specified operating parameters during its life cycle. The life cycle of an item comprises Concept, Research & Development, Production, Operation & Maintenance and, Disposal phases. Each phase carry costs its owner wishes to minimize. The idea is to realize the most value from the item when the whole life cycle costs and benefits are considered. In most cases, usually 80% of the total costs are incurred during the operation & maintenance phase of the life cycle. Machine failures cause plants to stop production causing accidents, economic impacts and reputation loses. Asset components gradual degradation with age, operational/maintenance errors and design flaws all can cause assets or processes to fail. A failed asset is considered unreliable, which means that it is no longer able to fulfill its intended function.
Reliability is defined as the ability of an asset to perform a specific function under stated operating condition for a specified period of time. Reliability is also a design characteristic elected by asset owners to fulfill specific operating conditions meeting business objectives. Reliability depends on item design, components quality control, manufacturing processes, and maintenance skills. Reliability is quantifiable and can be expressed in several significant ways depending on the purposes of the analysis. Reliability is related to maintenance and can be measured in terms of how often a component needs maintenance (fails). Reliability is the probability that a component or process will perform a specific function for a specified operating interval under a definite set of conditions too.
Reliability Engineering deals with the application of engineering principles and techniques throughout an item lifecycle. The goal of reliability engineering is to evaluate the inherent reliability of asset components and processes to identify potential areas for reliability improvement. Reliability engineering applies technology and methodologies to identify the most likely failures and recommends appropriate actions to mitigate their effects. Reliability analysis provide knowledge on items’ predominant failure characteristics helping maintainance engineers to choose appropriate tasks to regain reliability.
Reliability, Availability, and Maintainability (RAM) Analysis
Mean Time to failure (MTBF) is considered a reliability parameter in RAM analysis. By the same token, Mean Corrective Time (Mct), often known as MTTR (mean time to repair), is the quantitative parameter related to maintainability, and it is measured in repair time. Maintainability is a design characteristic of assets dealing with ease, accuracy, safety, and economy in the execution of maintenance functions. Equations X.1 and X.2 express how MTBF and Mct are calculated, respectively. It is observed from Equation X.3 that both reliability and maintainability influence the Inherent Availability (Ai) of assets.
operating time
MTBF = ____________ (X.1)
number of failures
repair time
Mct = ____________ (X.2)
number of failures
MTBF
Ai = ____________ (X.3)
MTBF + Mct
Many companies today define Ai expectations for plant systems. Then, plant designers must take this information into consideration when selecting systems configurations enabling proper design characteristics yielding the necessary reliability and maintainability performance for the required Ai. RAM analysis enables reliability analysts to define assets’ reliability, maintainability, and resulting availability under their current operating context. Actions to improve maintainability or reliability can be taken if the current system’ s availability is not acceptable to its owner. Vital information is drawn from both corrective and proactive work orders for determining these important quantitative parameters. The need for better data becomes a real issue when a company wishes to improve its operational yield by optimizing the operation’ s availability for increased profitability. Keeping RAM parameters as key performance indicators (KPIs) is not difficult once appropriate data is there in the work orders for its calculation. RAM analysis is quite easy to calculate. RAM analysis is also flexible, as it can be applied to a single asset or to a whole system by simply including in the mathematics all failure events of the desired system over a defined period of time. RAM analysis has some limitations, being based on average data. Both repair times and time between failures calculations yield average data that is fit for the purpose of the analysis. Analyzing average data alone could, however, be misleading. The use of averages may mask the actual predominant failure patterns and lead to misapplication of consequence management policies.
Failure Data Analysis
Statistical life data analysis considers reliability as a probability of fulfilling a specified function instead of an average time to failure of an item, as is the case in RAM analysis. The analysis is particularly applicable to assets with operating and maintenance history with well-documented failure events. Such events should be recorded and sorted by failure causes. Some of the outcomes and applications of life data analysis are the following:
- Failure forecasting and prediction
- Evaluating corrective action plans
- Test demonstration for new designs with minimum cost
- Maintenance planning and cost-effective replacement strategies
- Spare parts forecasting
- Warranty analysis and support cost predictions
- Controlling production processes
- Calibration of complex design systems
- Recommendations to management in response to service problems Mechanical, electrical, electronic, material, and even human failures can be modeled and predicted using failure data analysis techniques, as can other deficiencies related to quality control and design issues.
- Determining reliability and probability of failure at any operating time
- Determining the item’ s predominant failure patterns (physics of the failure)
- Confirming appropriate consequence management strategies selection in RCM analysis
- Calculating time-based task frequencies
Weibull Analysis
Basic Weibull analysis consists of plotting failure data on Weibull probabilistic paper and
interpreting the plot. Weibull plots are found to be very effective with extremely small samples of data for engineering analysis of even two or three data points. Predictions of failures and their corresponding costs, spare parts consumption, labor usage, failure rates, and electrical outages can be determined accurately through the use of this magnificent statistical tool.
In Weibull analysis, the reliability function R(t) corresponding to the probability that an item survives to any given age. R(t) is the probability that the failure does not occur in the interval o to t. Then,
F(t) is the cumulative distribution and represents the probability of failure at or before
operating age t. Then,
Creating and Interpreting Weibull Data Plots
The major advantage of Weibull analysis is its ability to provide accurate failure analysis and forecasts with extremely small samples. Our asset management efforts to stem incidents of critical machinery failing can benefit from such analysis, which reveals the nature of the failure patterns being experienced. Predominant failure patterns, failure probabilities, consequence management policies, and optimum replacement times can be easily determined at the failure cause level. Another advantage of the method is that it provides a simple graphical plot of the failure data, which can be easily interpreted, somewhat intuitively, without the need for any calculation.
Weibull data plotting entails the graphing of failure time versus probability of failure on a particularly designed logarithmically scaled Weibull probabilistic paper. Therefore, it is a log plot of F(t), for which the horizontal scale of the plot is a measure of life or aging by the use of a time parameter (t). Life data means that we need to know the age of the items failing and in service. The time parameter t can be expressed in mileage (for vehicles), operating time, operating cycles, starts and stops, landings, takeoffs, storage time, etc. The best aging parameter is the one with the best fit compared with a straight line in the Weibull plot. The vertical scale represents the cumulative percentage of failed items or the probability of failure F(t) up to time t. The X-axis plotting position corresponds to the age at failure. The Y-axis plotting position is the probability of failure value.
The defining parameters of the Weibull line are the shape parameter β and the characteristic life or scale parameter η. β offers an idea of the physics of the failure that the item exhibits, such as infant mortality, random, or wear out. It equals the slope of the Weibull plot line on the Weibull plot.
The scale parameter η, also called the characteristic life, equals the time for a probability of failure of 63.2% for every value of β.
Weibull plots are constructed either manually or through the use of software. Figure X.1 shows a Weibull plot for 3 failure events of a particular failure mode. The shape parameter β equals the slope of the Weibull plot line (3.65 in this case). Also, the scale parameter η is easily obtained by inspecting the graph for the time corresponding to a 63.2% probability of failure. A horizontal dotted line crosses the graph exactly at 63.2%, and its intercept with the plot line yields a characteristic life of approximately 445 h. Unreliability values for any age are determined by inspection of the Weibull plot as was done for the β and η parameters. For example, F10 , or the time for which there is 10% probability of failure, corresponds to an operating time of approximately 238 h by inspection. Weibull analyses are carried out for single failure causes subject to unique operating contexts.
How do we interpret the Weibull plot?
The following table summarizes the relation of β values with the physics of the failure for each individual failure cause.
In this case, β > 3, meaning that we have a strong wear-out case, for which T-type tasks are recommended. The Weibull analysis suggests that a Time-Based Maintenance task be used to tackle this failure mode.
Reliability can be represented by MTBF in RAM analysis and as R(t) or probability for not failing in Weibull analysis. Weibull analysis involves the creation of statistical models from failure events data. While RAM analysis can be applied to single or combined failure modes, Weibull analysis is useful only when applied to specific failure modes with precise failure ages.
Both analyses are used by reliability engineers to improve asset reliability, availability and maintainability. Thus, REAM (Reliability Engineering Applied to Maintenance) deals with the use of quantitative reliability analyses for improving maintenance decisions on appropriate task and frequency interval selection, among other aspects.
Download course brochure in English or in Spanish.